Introduction
In recent years, Artificial Intelligence (AI) technology has made significant strides, transforming various industries and revolutionizing the way we live, work, and communicate. From smart assistants to predictive algorithms, AI systems have become essential tools that aid in decision-making and automate tasks. However, with such advancements, a new concern has emerged: AI hallucination. But what exactly is AI hallucination? How does it occur? And most importantly, how can we prevent it?
In this article, we’ll delve into the fascinating world of AI hallucination, exploring its nature, causes, and potential dangers. We’ll also provide effective strategies to ensure AI systems remain accurate and reliable, safeguarding us from the pitfalls of misinformation.
Understanding AI Hallucination
AI hallucination refers to the phenomenon where artificial intelligence systems generate inaccurate or misleading information. It occurs when AI models produce outputs that do not align with reality due to biases, incomplete training data, or unexpected patterns. Just like optical illusions deceive our visual perception, AI hallucination can mislead us into trusting false information.
Causes of AI Hallucination
AI hallucination can be caused by various factors:
Biased training data: If the data used to train AI models is biased or incomplete, it can result in skewed outputs. For example, if a language model is predominantly trained on texts from one demographic group, it may produce biased or discriminatory responses when interacting with other groups.
Contextual understanding limitations: AI systems may struggle to comprehend the context and nuances of human language, leading to misinterpretations. This can result in generating incorrect or nonsensical responses.
Lack of diverse training examples: AI models need exposure to diverse scenarios during training to ensure they can handle a wide range of inputs. As a result, without sufficient exposure, they may struggle to provide accurate outputs for unfamiliar scenarios.
Potential Risks and Impact
AI hallucination poses several risks that can have far-reaching consequences:
Misleading information: AI systems may produce seemingly plausible yet false or misleading information, deceiving users and causing them to make incorrect decisions based on inaccurate outputs.
Amplification of biases: If AI models are trained on biased data, they may perpetuate and amplify existing biases, leading to discriminatory or unfair outcomes. This can be particularly concerning in domains like hiring or lending decisions, where bias-free algorithms are crucial.
Loss of trust and credibility: Inaccurate AI outputs can undermine trust in AI systems. Hence, leading to skepticism and reduced reliance on this transformative technology. Regaining trust and rebuilding credibility can be challenging once lost.
Preventing AI Hallucination
Now that we understand the concept and potential risks of AI hallucination, let’s explore some actionable strategies to prevent its occurrence:
- Ensure Diverse and Balanced Training Data
To minimize biases and improve AI accuracy, it is crucial to use diverse and balanced training data. This entails incorporating different demographics, perspectives, and cultural nuances to foster a more comprehensive understanding of the world. By representing a broad range of experiences, AI models can provide more impartial and equitable outputs.
- Continual Evaluation and Iteration
Regular evaluation of AI models is essential to identify and rectify any hallucination tendencies. Perform thorough testing on various inputs to uncover potential biases, verify outputs for accuracy, and assess AI system performance. Implement an iterative process to refine the model, addressing any identified issues, and improving overall reliability.
- Incorporate Explainability and Transparency
Enhancing AI systems’ explainability and transparency can help users understand the reasoning behind AI-generated outputs. By providing clear explanations or visualizing the decision-making process, users can better evaluate AI suggestions and identify potential flaws or biases. This fosters trust and empowers users to make informed decisions.
- Regularly Update and Retrain AI Models
As our understanding of the world evolves, AI models should be updated and retrained to adapt to changing circumstances. Stay vigilant in keeping up with advancements in research, data, and best practices to ensure AI systems remain accurate, up-to-date, and aligned with societal values.
- Collaboration and Ethical Guidelines
Promote collaboration between AI researchers, policymakers, and domain experts to establish ethical guidelines and standards for AI development and deployment. Furthermore, encourage interdisciplinary discussions to address potential bias, ethics, and societal impacts, allowing for collective responsibility in shaping AI systems that benefit all.
FAQs
Can AI hallucination occur in specific domains, such as natural language processing or computer vision?
Yes, AI hallucination does not limit itself to any particular domain. It can occur in various AI applications, including natural language processing, computer vision, and decision-making algorithms.
Are there any known instances of AI hallucination negatively impacting real-world scenarios?
While there have been reports of AI systems producing misleading or biased outputs, the impact in real-world scenarios is not yet widespread. However, proactive prevention measures are essential to mitigate potential risks as AI becomes more prevalent.
Are AI hallucinations intentional or unintentional?
AI hallucinations are typically unintentional and stem from biases, limitations in training data, or the AI system’s contextual understanding. Developers aim to create dependable AI systems, but unforeseen hallucinations can occur during complex interactions.
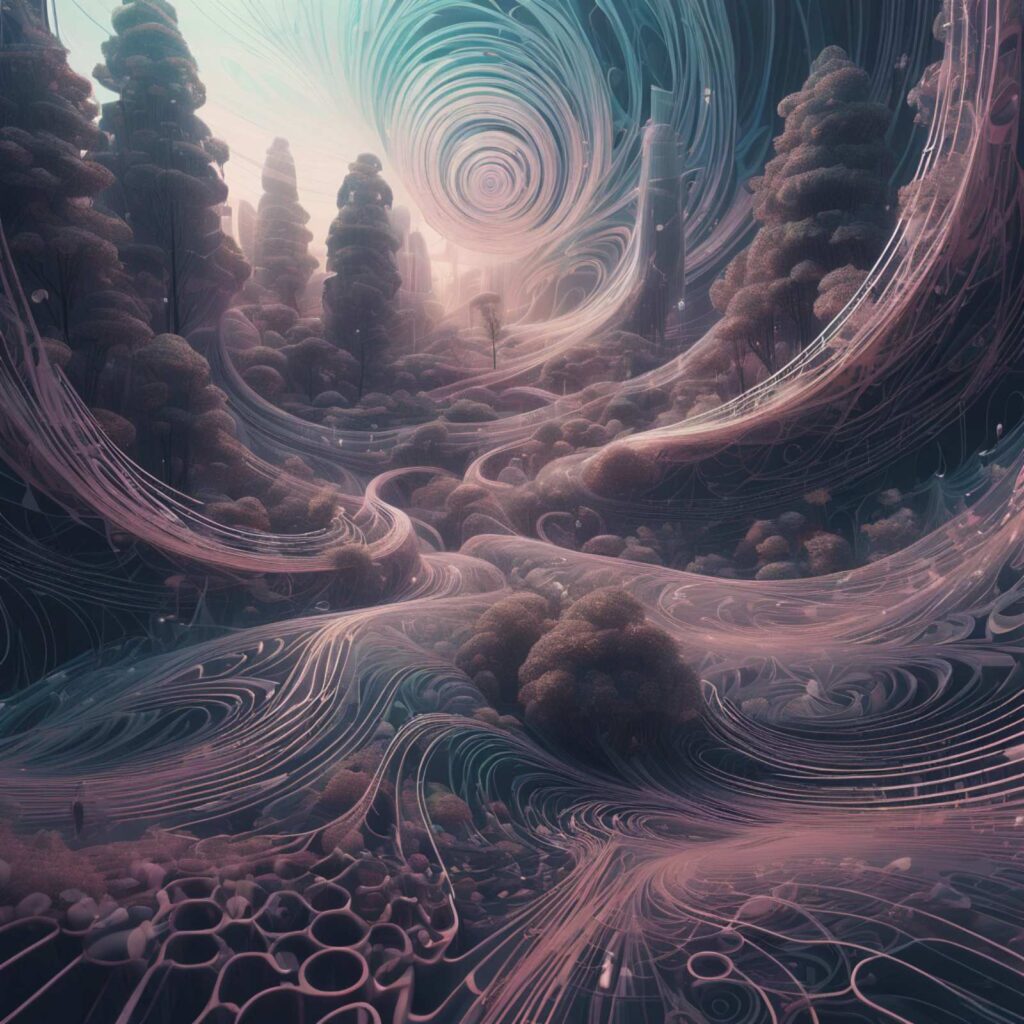
Conclusion
As AI technology continues to advance, it is paramount to address the challenges posed by AI hallucination. By understanding its causes, potential risks, and applying effective prevention strategies such as ensuring diverse training data, continual evaluation, and transparency, we can maintain AI accuracy and reliability. Encouraging collaboration and establishing ethical guidelines will further ensure AI systems promote fairness and inclusivity. Let us forge ahead, armed with knowledge and proactive measures, to shape a future where AI benefits us all without the burden of hallucination!